Peking University student Wang Di wins 2019 Data Mining Best Paper Competition
Nov 29, 2019
Peking University, Nov. 26, 2019: The 2019 INFORMS Annual Meeting was held from October 19 to 23 in Seattle, USA. Wang Di, a Ph.D. candidate from the Department of Industrial Engineering and Management, College of Engineering, Peking University, won 2019 Data Mining Best Paper Competition of this conference.
Wang Di (right)
The INFORMS Annual Meeting is organized by the International Association of Operations Research and Management Sciences (INFORMS) and is held annually in North America. It is a top academic event in the field of operations research and management science. The 2019 INFORMS Annual Meeting attracted more than 6,000 INFORMS members, students, prospective employers and employees, and academic and industry experts who compose the INFORMS community.
The INFORMS Data Mining Best Paper Competition, which was launched more than ten years ago, attracts scholars and doctoral students from top universities around the world each year. The winners of the competition are young scholars who have achieved outstanding research results in this field and demonstrated great development potential.
This year, the competition received high-quality academic papers from more than 70 research groups around the world. After rigorous selection by the awards committee, four academic papers from Massachusetts Institute of Technology, Georgia Institute of Technology (ranked No.1 globally in industrial engineering for 27 consecutive years), Northwestern University (ranked No.4 in the United States), and Peking University were nominated for the final contest. In the end, Wang Di stood out with outstanding performance and became the only winner. This is the first time that a student from a university on the Chinese mainland has received this honor.
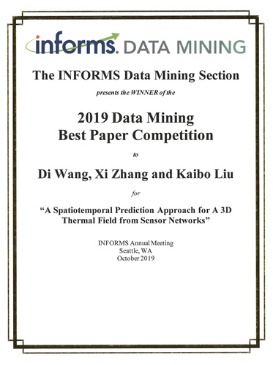
Award certificate
Wang Di's award-winning paper is entitled "A Spatiotemporal Prediction Approach for a 3D Thermal Field with Sparse Data". This paper fully considers the temperature-temporal dynamic coupling characteristics of engineering systems and the problem of missing temperature sensor data. Using a combination of data analysis and dynamic modeling techniques, a joint model based on thermodynamics and space-time transfer learning is proposed. Based on the actual situation of China's grain reserve depot, she took into account the temperature sensor data of multiple engineering systems with similar attributes to predict the target temperature system, which has a wide application in engineering service systems.
The results of this research have been developed into a software and used as a pilot application in China's grain reserve monitoring system. The paper was highly recognized by the awards committee. Professor Evangelos Triantaphyllou from Louisiana State University, chairman of the awards committee, said the paper has important theoretical value and application prospects.
Written by: Kang Meiling
Edited by: Ma Yaoli
Source: PKU News (Chinese)